What is the potential of hyperspectral retinal imaging?
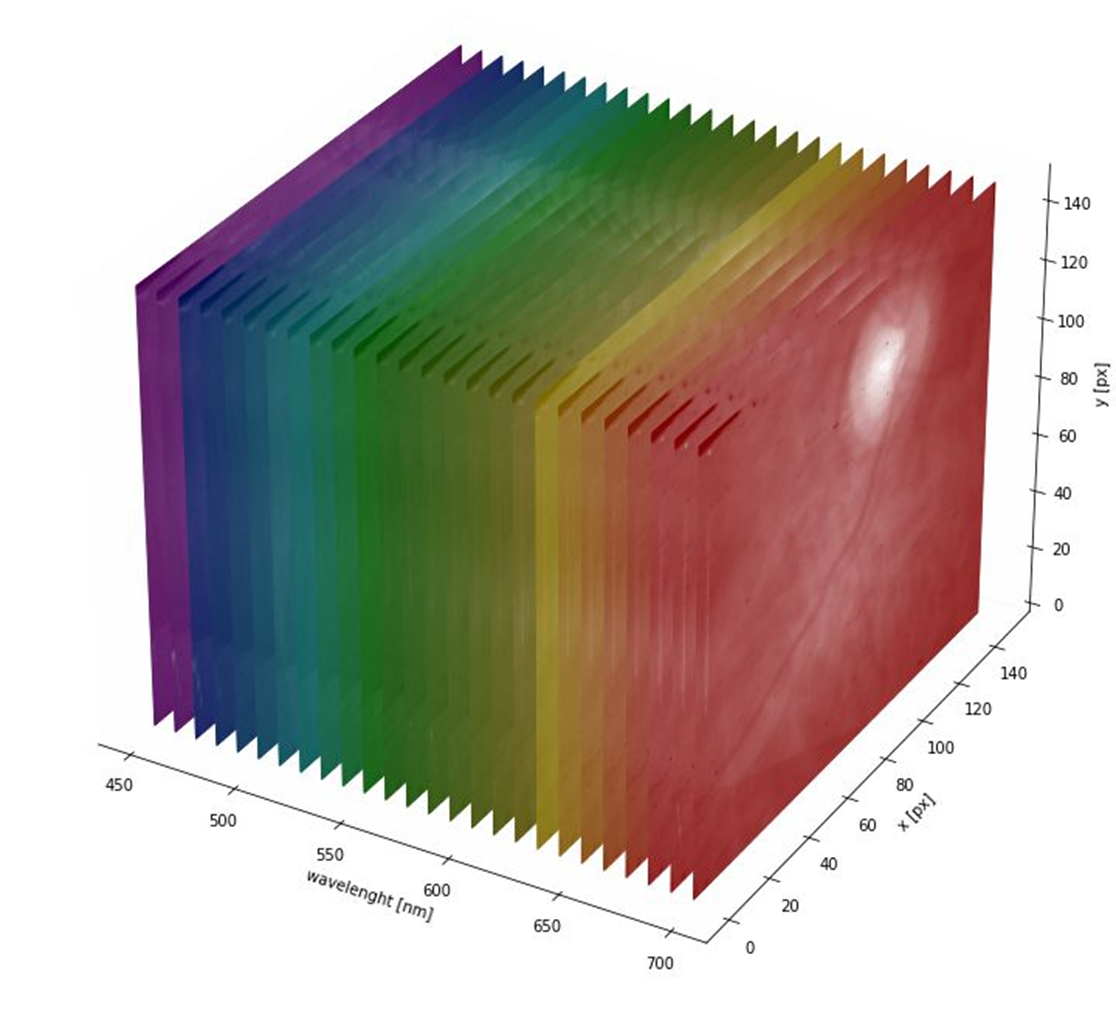
In 2016, Google has published a deep learning algorithm to detect diabetic retinopathy (DR) called Automated Retinal Disease Assessment (ARDA)¹. The model trained on more than 100,000 retinal images was able to “identify key markers of diabetic retinopathy, such as nerve tissue damage, swelling, and haemorrhaging.” and had a 97.5% sensitivity and 93.4% specificity in diagnosing DR.
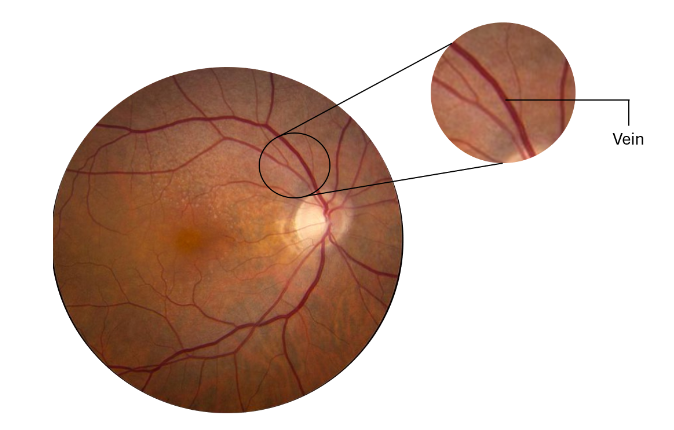
This model represented a revolution in the field of ophthalmology as it scaled up the detection of diabetic retinopathy. This is particularly important since the 420 million people with diabetes worldwide are at risk of developing diabetic retinopathy and almost 2 million people are blind because of it. Early screening of DR allows for simple and effective prevention measures to be taken and treatment to be more effective. Today ARDA is being used to detect DR in India and the European Union with almost 3000 new screenings supported by ARDA weekly.
However, this model has two limitations:
- It can only detect the disease once the damages are already present and therefore too late to prevent them.
- There are other diseases (AMD, Glaucoma) that also causes millions of cases of blindness.
This is where hyperspectral imaging comes into play, indeed this technology initially developed for earth observation allows to measure the chemical composition of the retina, for example the oxygenation of the blood or the presence of chemicals which are either protective (macular pigment) or toxic (lipofuschin).
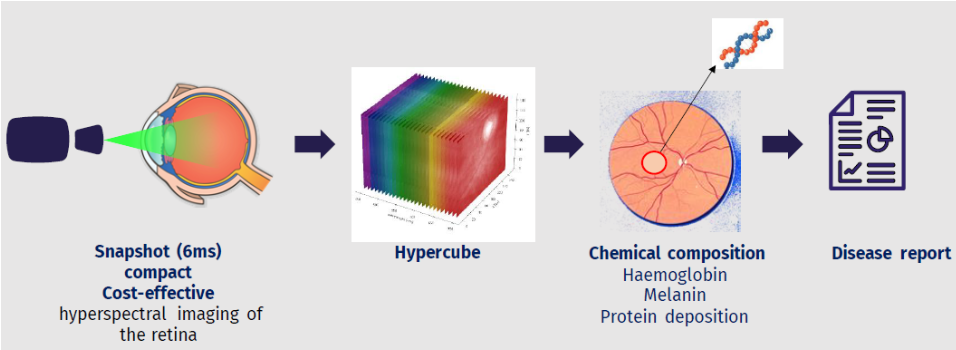
Such information is extremely valuable as it allows to see if a patient is at risk to develop a disease before any damages occurs, thus increasing considerably the time window where prevention and treatments are effective.
¹Ref: V Gulshan, L Peng, M Coram, MC Stumpe, D Wu, A Narayanaswamy, etal, Development and validation of a deep learning algorithm for detection of diabetic retinopathy in retinal fundus photographs JAMA (2016).